Summary
Business intelligence has grown beyond its initial manifestation as dashboards and reports. In its current incarnation it has become a ubiquitous need for analytics and opportunities to answer questions with data. In this episode Amir Orad discusses the Sisense platform and how it facilitates the embedding of analytics and data insights in every aspect of organizational and end-user experiences.
Announcements
- Hello and welcome to the Data Engineering Podcast, the show about modern data management
- When you’re ready to build your next pipeline, or want to test out the projects you hear about on the show, you’ll need somewhere to deploy it, so check out our friends at Linode. With their new managed database service you can launch a production ready MySQL, Postgres, or MongoDB cluster in minutes, with automated backups, 40 Gbps connections from your application hosts, and high throughput SSDs. Go to dataengineeringpodcast.com/linode today and get a $100 credit to launch a database, create a Kubernetes cluster, or take advantage of all of their other services. And don’t forget to thank them for their continued support of this show!
- Atlan is the metadata hub for your data ecosystem. Instead of locking your metadata into a new silo, unleash its transformative potential with Atlan’s active metadata capabilities. Push information about data freshness and quality to your business intelligence, automatically scale up and down your warehouse based on usage patterns, and let the bots answer those questions in Slack so that the humans can focus on delivering real value. Go to dataengineeringpodcast.com/atlan today to learn more about how Atlan’s active metadata platform is helping pioneering data teams like Postman, Plaid, WeWork & Unilever achieve extraordinary things with metadata and escape the chaos.
- Prefect is the modern Dataflow Automation platform for the modern data stack, empowering data practitioners to build, run and monitor robust pipelines at scale. Guided by the principle that the orchestrator shouldn’t get in your way, Prefect is the only tool of its kind to offer the flexibility to write code as workflows. Prefect specializes in glueing together the disparate pieces of a pipeline, and integrating with modern distributed compute libraries to bring power where you need it, when you need it. Trusted by thousands of organizations and supported by over 20,000 community members, Prefect powers over 100MM business critical tasks a month. For more information on Prefect, visit dataengineeringpodcast.com/prefect.
- Data engineers don’t enjoy writing, maintaining, and modifying ETL pipelines all day, every day. Especially once they realize 90% of all major data sources like Google Analytics, Salesforce, Adwords, Facebook, Spreadsheets, etc., are already available as plug-and-play connectors with reliable, intuitive SaaS solutions. Hevo Data is a highly reliable and intuitive data pipeline platform used by data engineers from 40+ countries to set up and run low-latency ELT pipelines with zero maintenance. Boasting more than 150 out-of-the-box connectors that can be set up in minutes, Hevo also allows you to monitor and control your pipelines. You get: real-time data flow visibility, fail-safe mechanisms, and alerts if anything breaks; preload transformations and auto-schema mapping precisely control how data lands in your destination; models and workflows to transform data for analytics; and reverse-ETL capability to move the transformed data back to your business software to inspire timely action. All of this, plus its transparent pricing and 24*7 live support, makes it consistently voted by users as the Leader in the Data Pipeline category on review platforms like G2. Go to dataengineeringpodcast.com/hevodata and sign up for a free 14-day trial that also comes with 24×7 support.
- Your host is Tobias Macey and today I’m interviewing Amir Orad about Sisense, a platform focused on providing intelligent analytics everywhere
Interview
- Introduction
- How did you get involved in the area of data management?
- Can you describe what Sisense is and the story behind it?
- What are the use cases and customers that you are focused on supporting?
- What is your view on the role of business intelligence in a data driven organization?
- How has the market shifted in recent years and what are the motivating factors for those changes?
- Many conversations around data and analytics are focused on self-service access. what are the capabilities that are required to make that a reality?
- What are the core challenges that teams face on their path to designing and implementing a solution that is comprehensible by their stakeholders?
- What is the role of automation vs. low-/no-code?
- What are the unique capabilities that Sisense offers compared to other BI or embedded analytics services?
- Can you describe how the Sisense platform is implemented?
- How have the design and goals changed since you started working on it?
- What is the workflow for someone working with Sisense?
- What are the options for integrating Sisense with an organization’s data platform?
- What are the most interesting, innovative, or unexpected ways that you have seen Sisense used?
- What are the most interesting, unexpected, or challenging lessons that you have learned while working on Sisense?
- When is Sisense the wrong choice?
- What do you have planned for the future of Sisense?
Contact Info
Parting Question
- From your perspective, what is the biggest gap in the tooling or technology for data management today?
Closing Announcements
- Thank you for listening! Don’t forget to check out our other shows. Podcast.__init__ covers the Python language, its community, and the innovative ways it is being used. The Machine Learning Podcast helps you go from idea to production with machine learning.
- Visit the site to subscribe to the show, sign up for the mailing list, and read the show notes.
- If you’ve learned something or tried out a project from the show then tell us about it! Email hosts@dataengineeringpodcast.com) with your story.
- To help other people find the show please leave a review on Apple Podcasts and tell your friends and co-workers
Links
The intro and outro music is from The Hug by The Freak Fandango Orchestra / CC BY-SA
Sponsored By:
- Linode: 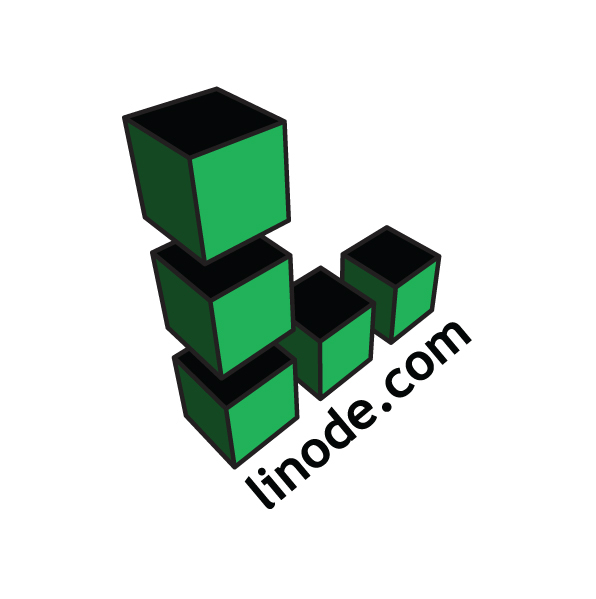 Your data platform needs to be scalable, fault tolerant, and performant, which means that you need the same from your cloud provider. Linode has been powering production systems for over 17 years, and now they’ve launched a fully managed Kubernetes platform. With the combined power of the Kubernetes engine for flexible and scalable deployments, and features like dedicated CPU instances, GPU instances, and object storage you’ve got everything you need to build a bulletproof data pipeline. If you go to: [dataengineeringpodcast.com/linode](https://www.dataengineeringpodcast.com/linode) today you’ll even get a $100 credit to use on building your own cluster, or object storage, or reliable backups, or… And while you’re there don’t forget to thank them for being a long-time supporter of the Data Engineering Podcast!
Hello, and welcome to the Data Engineering Podcast, the show about modern data management. Atlan is the metadata hub for your data ecosystem. Instead of locking your metadata into a new silo, unleash its transformative potential with Atlan's active metadata capabilities. Push information about data freshness and quality to your business intelligence, automatically scale up and down your warehouse based on usage patterns, and let the bots answer those questions in Slack so that the humans could focus on delivering real value. Go to data engineering podcast.com/atlin today, that's a t l a n, to learn more about how Atlan's active metadata platform is helping pioneering data teams like Postman, Plaid, WeWork, and Unilever achieve extraordinary things with metadata.
When you're ready to build your next pipeline or want to test out the projects you hear about on the show, you'll need somewhere to deploy it. So check out our friends at Linode. With their new managed database service, you can launch a production ready MySQL, Postgres or MongoDB cluster in minutes with automated backups, 40 gigabit connections from your application hosts, and high throughput SSDs. Go to data engineering podcast.com/ linode today and get a $100 credit to launch a database, create a Kubernetes cluster, or take advantage of all of their other services. And don't forget to thank them for their continued support of this show. Your host is Tobias Macy. And today, I'm interviewing Amir Orad about Sisense, a platform focused on providing intelligent analytics everywhere. So, Amir, can you start by introducing yourself? Thanks for having me, Amir Ohrad, Sisense chief exec. And do you remember how you first got started working in data?
[00:01:45] Unknown:
I've been doing this for more than 20 years. I feel old when I say that. But my previous 2 startups, both enriched critical mass, 1 was using data and real time risk engines to make cybersecurity decisions. And, actually, every time you log in to your bank account or Facebook and you get this text message with a code to put in, That's actually my invention. My name is on the patent still. We're using for the first time real time data analytics to make decisions. Do we send you that text message or not? That was 7 years of my life. And then I ran a company doing financial crime detection using big data to find patterns in banking activities to catch money laundering and cyber hacking and insider trading and human trafficking only by looking at data and catching the bad guys. And that was 8 years of my life literally going through every second financial transaction on the planet.
So that's 15 years of my life. Before that, I was a developer also building big data systems. So you can see the pattern here. 8 years ago, I met the Sisense team. I wasn't the founder, but I joined early on as the CEO and fell in love because they built a platform. I realized I had to do it myself in my previous companies all from scratch, like, literally button up. And I saw a platform that with the right APIs can actually help other companies, other entrepreneurs leverage data better. Fell in love, and 8 years, I'm still here.
[00:03:16] Unknown:
And so in terms of the Sisense platform, can you describe a bit about what it is and as much of the story behind it as your privy 2?
[00:03:25] Unknown:
Yeah. Definitely. So we are in the BI space or the analytics space. And in theory, it's a valve space with many names like Power BI and Tableau, etcetera. However, we're the only company out there that is doing and focusing only on 1 thing, embedding analytics via APIs, embedding analytics into other people applications, internal applications, external applications mostly, etcetera. And we found that when we started, it was a small niche, but now more and more companies, about 2, 000 today, already leverage us because we believe that every application on the planet will become data driven with analytics built in, visual analytics, and service the users with analytics or become obsolete.
And in the past, you used to have a operational system, can be in a bank or in a pizza shop or in a telco. It doesn't matter. And next to it, like side by side, you would have your analytical tool. Business objects back in the day and SAP and Oracle, and then it evolves to Tableau and Power BI and Luca. Those were separate systems. And what we saw is when you have separate environments, you have much less adoption. You look at things out of context and it is not aligned with the future, which is all API based, cloud based, modern datasets, etcetera. So that's how it started.
And we built the company API first, bottom up, while most analytics tools are built for a business analyst first. So we optimize for the integration. Other people optimize for the analysts. And still we have to service the end users who are not analytical experts. They are doctors and nurses and truck drivers and, you know, teachers, etcetera, that are using those applications we are part of. So that's how we evolved. I'll give you 2 basic examples just to make it, you know, tangible. We are part of nasdaq.com. And when a CFO of a public company wants to analyze who's trading their stock, they they go to nasdaq.com/insights, and that entire Insights experience is running on Sisense. So we bring the data from the Nasdaq datasets, massage it, run the analytical queries, put visualizations on top, all API integrated into the nasdaq.com application, and a CFO can consume that as part of their Nasdaq relationship and not as a separate tool. You don't have to download the data to a CSV file, take it elsewhere, and use it. That's just a small example and maybe a totally different example, but 1 I love because it saves lives.
For anyone who's been in San Francisco lately, if you go on the Golden Gate Bridge literally, as you get on the bridge, there's a big sign that says, if you have suicidal thoughts, call this number, 1800 text line. And they take your call and help you literally get off the ledge, I guess, and not have those bad thoughts. When they are on the phone with you behind the scenes with a machine learning system that using Sisense to recommend to the agent what's the best thing to say given time of day, weather, demographics, historical behavior, etcetera, it's part of the experience. It's not a separate tool that after the fact 1 day will give you a report.
It's contextual to that experience. So 2 very different examples, both embedded to an application that service the users of that application, in this case, external applications. But may hopefully gives you a feeling to what we do.
[00:07:05] Unknown:
So in terms of the, I guess, use cases and audience types that you're focused on, I'm wondering if there are any kind of broad categorizations or generalizations that you can make, or if it's just a matter of you kind of build the platform and everybody who comes to it comes to it for their own reasons?
[00:07:26] Unknown:
It's an excellent question. We do see some verticals more than others, mainly the data rich verticals in healthcare, financial services, tech companies, obviously logistics and supply chain companies, but we also have the United Nations and other the Red Cross's customers that suits really wide. But there's something common to each and every example, and that is the entity we work with sits on very interesting datasets in 1 location or many, and the data team or the product team or the engineering team wants to leverage that data and create insights inside their end user facing application.
And that's always a pattern. So we work with the data team, data engineers, data scientists, etcetera, product people, which are the technical people and the product people that build the application, and then the business people that want to service their audience internal or external. That pattern is identical if you're a supply chain company, if you are, NASDAQ, or if you are Philips Medical Devices, another example. It's the exact same set of people we work with and the exact same outcome we want to achieve. You mentioned that the, I guess, broad category
[00:08:43] Unknown:
that people put you into is that of business intelligence, and that's a category that has been seeing a lot of I don't know if identity crisis is the right term, but I've been seeing a lot of kind of change and evolution from what people traditionally think of as business intelligence that, you know, as it existed in the nineties early 2000. And I'm wondering what you see as the current working definition of what business intelligence is or should be and some of the market shifts and motivating factors for the evolution from where that term originated to where we are today.
[00:09:18] Unknown:
You know, back in the day, when I was younger, there used to be Internet companies. Maybe you recall the day where Internet companies and companies and non Internet companies, and that's not the case anymore. There's Internet companies and dead companies. Everyone are Internet companies. Everyone leverage the Internet. I think BI as a category would almost will become analytics, but business intelligence will be in everything. So everything will be leveraging business intelligence because when you watch Netflix, there's business intelligence telling you which next movie to watch. Right? So those technologies are appearing everywhere.
So that's the broad category as it was defined in the past. I think what it's becoming is now a set of analytical engines that leveraging modern cloud and API technologies can be integrated into and inserted into every application out there as more fundamental pipes, if you like, or infrastructure. And that is where it's heading. Gartner has a new term called composable analytics and composable applications. What I mean by that is future applications will be composed of many little ingredients. Some of those ingredients will be the pipes, the data pipes. Some will be the databases.
Some will be the data analytics. Some will be the visualization. So you're moving from a BI kind of monolith to best in class components you can build applications from, and that's where we play. And I know Gartner actually said publicly they were debating, do they do embedded analytics as a category? But they said everything 1 day will need those skills, so it's not time to separate independent category. So that's a long answer to say, I think, a, all companies will become data driven or obsolete, and, b, business intelligence will become analytical components you integrate into other applications versus separate stand alone on the side tools that only a few selected people can use.
[00:11:24] Unknown:
Another interesting aspect of the kind of business intelligence category is that it's intended to be directed towards end users, people who don't necessarily identify as technical. And so the kind of way that it was generally approached or manifested is as this self-service interface to the broader data ecosystem where it's kind of, here is your playground where it's safe for you to do things and ask questions, but if you wanna do anything more complex, then you're gonna have to ask somebody else for help. And I'm wondering what you see as the kind of table stakes for self-service access to organizational data and the ways that business intelligence needs to be kind of adapted or evolved to be able to fit that requirement?
[00:12:12] Unknown:
Yeah. It's an excellent question. I think there was a lot of progress, but also a lot of hype around this democratization of business intelligence. We did move while a decade ago, 15 years ago, IT was owning the data and and you have to be an IT person to really understand what to do with it. We did make a big jump forward and Tableau gets the credit early on to enable and democratize business analysts with data. So we moved from IT only to IT and business people and business analysts. However, we are almost at the end of 2022, early 2023, and still 80% of the people in organizations don't use BI because it's built for business analysts.
And most people in the company are factory workers and doctors and nurses and logistics managers and HR people and marketing people and all of those things. And we're not 1 thing. We're not BI experts. We don't want to be. We don't care about BI. We just want answers. And that's what I feel is propelling companies like Sisense, which I didn't mention. But for context, we're not a startup anymore. We have, you know, over a 100, 000, 000 business in size and thousands of clients because to address the 80%, they don't want tools for BI people. They want tools for people, human beings, nothing about technology.
And that's the unserved part of the market. And that promise has not been met yet. We democratized only for the BI experts. And that's why you get natural language technologies, augmented analytics, AI, and embedded, what I'm talking about, all coming to play to enable those people that are not experts.
[00:14:05] Unknown:
As far as the kind of self-service data doc democratization, what are some of the, I guess, organizational or infrastructure challenges that teams are having to struggle with or some of the technical, either capabilities or investments that need to be grown or established to be able to support that kind of organization wide capability?
[00:14:32] Unknown:
There are 2 approaches today that are common, which are the old way of doing things, but the current 1 is there is a central BI team or departmental BI team who builds on behalf of the human beings dashboards. For example, if I'm a teacher and I want to know how my students are doing, I have to go to a separate application, log in, and hope that my BI expert in the school built in advance an answer to my question. That's like approach number 1. If it's the company is less advanced, it will be a central big dashboard with many, many things for all employees in the company. Small advance would be someone in the teacher's area that builds the dashboard.
Option number 2 is that I will be offered access to tools that if I'm really savvy, will allow me to ask a question on the fly using some drag and drop dimensions and data and time horizon and, you know, hopefully understand correlations and regressions and can connect the things together and find out how my students are doing. And those are the 2 current main approaches. Then we believe with the 3rd approach, which is as a teacher, when I use my day to day teaching application where I give homework and grades and everything, there'll be another indicator next to every student name with a red flag, yellow flag, green flag saying how they're doing.
And I'll get an alert practically without asking any questions saying, did you know that you have 2 students who suddenly dropped in their performance? You should take a look. That's I believe the future of analytics may come to you. They are in the tools you already use. They're not some separate thing, and they are natural to the context you're in. And I believe that's the next level as an industry should push towards because I'm more likely to use it as a teacher. And my student is more likely to be saved from dropping out of school if I get this alert and this flag versus me chasing some dashboard somewhere.
[00:16:37] Unknown:
To that point of the kind of level of understanding required for that second approach of here are all the tools. Good luck. Go ahead and figure it out. That can potentially lead to the issue of, I found an answer, and I'm happy with the answer, but it's actually the wrong 1. And I'm wondering what are some of the ways that as tool builders, as, you know, companies providing platforms, how can we work towards adding some useful guardrails and improving that user experience of, okay, you want to be able to get to this answer. We're going to help you understand the different kind of joins or the different ways to explore the data that is accurate and actually provides a safe and reliable answer.
And, you know or even just to the point of helping people understand, like, what are some of the viable answers or being able to work at the level of their understanding of saying, like, this is the thing that I want to do. Like, I want to understand how my students are doing in terms of their grades. And then saying, okay. Here are all the different tables that you need to work across. Here are some different ways that you can join them.
[00:17:46] Unknown:
So I think what you just said, part of the question is part of the answer and the problem. A teacher that wants to know how the student is doing should not know a thing about joins and tables and views and indexes. Nothing. You shouldn't know it exists. You should ask a question and get an answer. Unfortunately, technology in the past limited us to forcing to know those concepts, fact tables and dimensions, etcetera. But in an ideal universe and the technology is making progress in that direction, they can ask a question in natural English and just get an answer.
Or even better, not ask a question, but be told the answer already. When you watch a movie on Netflix and us as consumers, I believe, is better analytics than us as professionals. You don't ask Netflix what's next movie I should watch. It just tells you. And if you finish an episode, you don't ask Netflix what's the next episode. It just offers it to you. And when I log into Amazon, I don't tell them it's my kid's birthday. They tell me, we saw you bought a lot of stuff for a party. Maybe you want this thing for the party. They answer the question before we ask them. Those are simple examples, and I would claim that an HR person that has done a merit cycle, the AI can guess if they want to know what's the distribution of merit and performance against merit and so on. And for patterns across many organizations, you can match.
So I believe that the answer is not teaching all of those things because with great powers come great responsibilities and we may get wrong answers and confuse people too often. But to build technology and tools that shortcut this process and never give answers proactively, or let them ask them in English with the intent and then do all the leg work behind the scenes. And the technology is very close to being there already, if not there already.
[00:19:46] Unknown:
Prefect is the data flow automation platform for the modern data stack, empowering data practitioners to build, run, and monitor robust pipelines at scale. Guided by the principle that the shouldn't get in your way, Prefect is the only tool of its kind to offer the flexibility to write workflows as code. Prefect specializes in gluing together the disparate pieces of a pipeline and integrating with modern distributed compute libraries to bring power where you need it, when you need it. Trusted by thousands of organizations and supported by over 20, 000 community members, Prefect powers over 100, 000, 000 business critical tasks a month. For more information on Prefect, go to dataengineeringpodcast.com/prefect today. That's prefect.
In terms of the end user experience, what do you see as the role of the, you know, current batch of low code and no code tools as a way to be able to make that interface more accessible and comprehensible to somebody who doesn't have an extensive background in analytics?
[00:20:51] Unknown:
That's an excellent point. That's really the bridge between the 2 realities we discussed. Right? So 1 reality, the teacher just wants an answer if he know nothing or joins. The other reality, a BI expert is building that query and dashboard for them. Local applications are creating a bridge between the 2, and they are necessary evil between current reality and the future technology that I think will really progress and advance the market. Not just in analytics, in general, in application development. There's not enough developers to build all the applications you want. So I expect to see more and more low code applications include basic analytical capabilities built in, simplified using the same low code concepts you use for other use cases.
I can tell you we built in Sisense, for example. We asked ourselves, how can you join tables by a local person? And we literally drag and drop 2 tables, connect them together, and and the computer guesses left join, right join, and the many to many complexities that's come with it as a way to bridge this reality from an expert to someone who know nothing. So I expect it to be a bridge. I think it's a good development in the industry. I think it can help democratize access to insights more. And like always, I think it will 1 day not be needed because the AI will just build you the application of the dashboards completely, and you can skip that phase a few more years before we get
[00:22:23] Unknown:
there. In terms of the Sisense platform itself, what are some of the unique capabilities or unique usage patterns that you target as far as this space of business intelligence, ubiquitous analytics, and being able to make those analytical capabilities more accessible to people who don't want to dedicate their time learning about, you know, what's the difference between the left, right, or inner join?
[00:22:49] Unknown:
So the 2 extreme areas we have invested in over a decade of innovation and research. Number 1 is developers and making it API first. So we have endless technology that allows that is all built above APIs, allows to integrate and embed Sisense into other people applications and products from, you know, GitLab integrated into their environment all the way to the ability to integrate Sisense inside Excel and PowerPoint, believe it or not, the output of Sisense Sisense. So that's developer tools from multi tenant technology to CD capabilities, from data APIs to security APIs, from query optimizations, what have you. Because if you want to integrate analytics into sound application, that's a very complex area to do well.
We found other tools in the market are doing that as a hobby, as a sideshow. It's not the main thing. And on the flip side, making it extremely easy to use because in a weird way, our users are less end users, not the BI admin or developer, but the end users are less of an expert less of ex being an expert in BI than typical users because they're the doctors and nurses and truck drivers and marketing people and the salespeople, etcetera. So we build technology that actually allows to look at 2 tables without having to understand joins, and natural language query, where you ask a question in English, and generation when you look at the dataset or the pie chart and it tells you in English what it means.
Predictive power. So for example, if you're not an expert in data, what we do is we take any time serious data and just predict the future without any action required by the user visually. And what we do behind the scenes, we need to have numerous over a dozen machine learning algorithms. We try on the data, AB test it, find the highest likelihood of success based on the future data, and pick that 1 and recommend it. So extreme ease of use on 1 end, using AI, predictive power, etcetera, and extreme API focus on the other end, which are 2 very different disciplines. And do all of that natively to cloud leveraging, you know, if you run on My Cloud, your Cloud, on GCP, on Azure, on Snowflake, on Redshift, on BigQuery, but also on CSV files, Hadoop, and, Oracle servers. So that breadth of integration points is really important because in real life, people want to believe they have 1 data warehouse that does everything, but usually the data is spread among different locations and different people, unfortunately.
[00:25:29] Unknown:
And as far as the implementation of the Sisense platform, what are some of the design and architectural aspects that you've leaned on to be able to power these use cases and be able to support this kind of ubiquitous integration across different downstream use cases and as well as working with some of the upstream data sources.
[00:25:51] Unknown:
So first, you mentioned the integration and architecture. It's really thinking in advance about the integration points and the architecture. Most analytical tool either run on a high performance database or require you to ETL and build pipes to do it via third party tool. And we found that to accelerate adoption, we have to support both. So we have our own in memory engine for data acceleration if it's not sitting on a Snowflake type technology, or you can build the pipes to Snowflake. If you have 1, it will sit on top and run queries on top. When I say Snowflake, I mean, all of those that layoff technologies. That's number 1.
We integrate to your CICD tools, your git repository. We allow you to run Python models on Sisense to take your machine learning models or statistical models and adjust analytics and put them on Sisense. So integration is key. I don't believe I can have the best set of tools I can integrate to the tools you use. And then architecture is to ensure this is all native cloud, Kubernetes, micro services, API first modern technology. So it has the agility and flexibility you need. So that's on that front. Instead of data, we are not replacing the need for data pipelines, but we can take the data from any part in that pipeline, depends how mature it is. As I mentioned, if you're fully mature, we'll take it from your Redshift or Snowflake Ubiquiti.
If you're not, we can take it from the source data, help you with data prep and acceleration of the data, and then get it into the engine. So that flexibility is really designed to drive agility because we found that if I'm part of someone's application, their application changes days and nights. And I need to be very agile to allow them to change with them and not slow down virginity. So those are some examples of concepts we have in the product. As you mentioned, the Sisense
[00:27:52] Unknown:
business and platform has been around for a number of years now. So obviously there have been a lot of shifts in terms of the underlying technologies or deployment patterns that you've been able to lean on as the surrounding ecosystem has grown and shifted to be very cloud oriented. I'm wondering what are some of the most notable evolutions or reimaginings that you've had to go through in terms of the overall goals and design of the platform?
[00:28:21] Unknown:
It's a really good question. I've said there are 3 areas that we had to reimagine. And reimagine, I'm the CEO, cost a lot of money also, but that's fine. It's a good investment. Number 1 was around the cloud. People adoption of the cloud in the data and analytics space up until 5 years ago was very low. People were terrified to leverage the cloud, even though other markets already did that. For security, privacy, governance, compliance, all sorts of reasons. And therefore, we've been forced to support a lot of on premise environments and, you know, Windows machines, etcetera.
We have reimagined and re architected everything about 7 years ago, 6 years ago, and built it from the bottom up leveraging the latest cloud Kubernetes technologies. That was a huge bet, but now we have 1 of the most modern application stacks or architectures out there as a result. That was number 1. Number 2 is the high performance databases or data warehouses. Up until Redshift and Snowflake and made it a big way kind of broke the barrier. Most organizations databases were not analytical in nature. In the SQL server, Hadoop, you know, the era of Hadoop and lakes, etcetera, but the compute was very slow. So we have to build those acceleration capabilities.
That has changed also in the last 5, 6 years with the Redshift, BigQuery, Snowflake, Methode, RISE that allows us to leverage those technologies and repeat native native integration already to r and d with those companies to create the ability to run analytics, send the query to that environment, and come back with the answer. That was the second 1. The third 1 is AI, AI machine learning. What we found I think we all know that the extreme rise in the capabilities available to people and data scientists and companies out there. And we decided to attack it in a weird way. We built 2 different set of tools, 1 for the data scientists that want to run their own models in Sisense, in Python and R, and we support that with the whole, you know, ID environment, etcetera.
So it's very integrated and that's typically not done inside analytical tools. On the flip side, we built our own AI out of the box functionality, like the prediction power I told you about focusing natural language because the simple end users need to also be serviced. So we use AI in 2 extremes for the savvy developers, data scientists, etcetera, and for the end users who know nothing about it and make it transparent. So those are the 3 areas I would say we have to really rebuild everything bottom up. The 1 area we did not have to do it is APIs. It was all built from APIs from the beginning.
By the way, not because we're geniuses, but because we have to compete in a very mature market. And the only way to do that is to create extensibility in the product for other people to build and integrate to. The APIs today are a massive asset. We have hundreds of APIs in the in the product that allow you to control everything. You don't have to see any UI. Everything from flow to adding users, from security to audits, from oversight to actual analytics and visualizations and acceleration. That has been and continues to be a core fundamental tenant in how we do things.
And I'm happy about that because the truth is, it's very hard to retroactively retrofit the technology to be API first. It's either is or it's not. I'm blessed by the fact that this team has built it the right way from the beginning.
[00:32:15] Unknown:
Another interesting aspect of the question of business intelligence and the analytics layer is that in addition to having gone through a bit of shift in terms of what the kind of identity and use cases are that it's supposed to support, it has also been starting to get subjected to the disaggregation that the database market has already gone through. And, you know, the pendulum has shifted a few directions in both ways, most notably in terms of things like security and access control, which was defaulting to the BI layer because that was where everybody interacted with the data. Now people are interacting in all kinds of different layers. The question of the kind of semantic or metrics layer of, you know, how do I define what this business or domain object happens to be? Is that something that lives in BI? Is that something that lives in my warehouse? You know, how do I define it? Where do I define it? Just in those dimensions, I'm curious how you think about the role of Sisense and the kind of layers of the data stack and the data life cycle at which people are kind of directed or encouraged to interact with Sisense and sort of what the role of Sisense is across that life cycle?
[00:33:25] Unknown:
That's a beautiful question, and I believe what we're doing is trailblazing. And I'll give you the basic example. CICD, version control. Today, the BI companies that support version control, some do not. Let's put them aside. That's really bad. Those who support it have our own version control component. But why? Why is a BI company an expert in building version control technology? So we took the opposite approach. We integrate to your Git repository with your Git tools, your CICD processes, your CICD workflows. Because I believe that if you build applications for a living and want to put analytics in it, you have the tools you already use and I should be part of Vend. So that's a great example. We're literally the only ones that we know of that are doing that in such way. You mentioned authentication and user management.
Yes. Technically, we have the ability to do our own user management, but we support 5 different kinds of SSO, integration of Okta and the like, user access tokens and tokenless and stateless environments and all sorts of chaos words, all designed to fit the tools you use in the applications you build. I mentioned before, I can leverage the snowflakes of the world when they exist and the Python models you build when it exists. But there, I let you leverage my technology because too many people don't have yet Snowflake or don't have yet data scientists to do the job.
So that's a place I'm still balancing my own out of the box functionality with APIs outside. 1 day, the pendulum will shift and everyone will have their own tools and I will integrate to them as the main workflow. So I'm a 1000% aligned with you and I believe we're it's a journey. And if you take to the extreme, BI companies will disappear and be turned into ingredients that are data ingredients, visualization ingredients, etcetera. And we're doing that at Sisense to ourselves. The company itself is built of different API components, which we believe is the future. So, yeah, we're very aligned on that view of the future.
[00:35:37] Unknown:
As far as the typical user interaction or the kind of onboarding process for somebody who wants to adopt Sisense and start using it for powering analytics, either for the traditional BI dashboard use case or embedding it into their applications. What does that kind of onboarding and user workflow look like, and how do the touch points of the Sisense platform shift across the different kind of data oriented and organizational oriented roles?
[00:36:09] Unknown:
Today, to consume Sisense or play around with it, you reach out to us and we give you a proof of technology environment to play with. And usually, it will be a specific project you want to demonstrate, you want to integrate into an application or build an application. We don't sell individual user licenses. We'll try to, for free, do that project with you. And then if it works, you know, have a real relationship, then have individuals trying to use it kind of it's a desktop tool. This is not a desktop tool. The use cases will be typically to integrate us into an internal application that is serving your employees.
For example, Northern Trust put us in for cash management application rebuilt or external application, for example, part of a medical service you give someone or part of a inventory management service you give someone or marketing service you give someone. We have people more and more trying to use us to integrate to productivity tools. So we have today native integration into Slack, Excel, PowerPoint, Google Sheets. This is where you consume the insights we generate into those productivity tools versus a set dashboard. Because, again, I believe that Inset should go to a URL, not as a site application. So if you only do a statement in PowerPoint, I'm a CEO. I develop in PowerPoint.
Then my PowerPoint can get insights from Sisense and, literally, have on the screen a number or a widget, a chart that comes from the Sisense server. So that's how you interact with us. The 1 use case I would tell you is not a good use for Sisense and I don't want to waste your time. If all you want is a generic dashboard that 10 different people can generate, I can do it. It's not the best way to use me. I'm sure there's cheaper tools out there that will do it for you even for free. But if you want to integrate, embed, make it part of an application, productivity tool, internal or external, we will shine and demonstrate the value to you and try it out.
Check how we do it. Go to sisense.com, fill the form, say you heard me and you want to check me out and see if I'm speaking the truth, try it out.
[00:38:20] Unknown:
Data engineers don't enjoy writing, maintaining, and modifying ETL pipelines all day every day, especially once they realize that 90% of all major data sources like Google Analytics, Salesforce, AdWords, Facebook, and Spreadsheets are already available as plug and play connectors with reliable intuitive SaaS solutions. Hivo Data is a highly reliable and intuitive data pipeline platform used by data engineers from over 40 countries to set up and run low latency ELT pipelines with 0 maintenance. Boasting more than a 150 out of the box connectors that can be set up in minutes, Hivo also allows you to monitor and control your pipelines. You get real time data flow visibility with fail safe mechanisms and alerts if anything breaks, preload transformations and auto schema mapping precisely control how data lands in your destination, models into workflows to transform data for analytics, and reverse ETL capability to move the transformed data back to your business software to inspire timely action.
All of this plus its transparent pricing and 247 live support makes it consistently voted by users as the leader in the data pipeline category on review platforms like g 2. Go to data engineering podcast.com/hebo data today and sign up for a free 14 day trial that also comes with 247 support. 1 of the other aspects that we haven't dug into much yet is the question of bringing machine learning into the analytical workflow where in a lot of cases, analytics and machine learning are seen as disparate or discrete activities where I have my data warehouse, I do some I do some transformations and I can provide analytics from that and somewhere else either from my data warehouse or my data lake, I will build features and generate my machine learning model and that has a different application.
And I'm wondering what you see as the intersection between analytics and ML and AI and some of the ways that we can start to bring machine learning and AI capabilities into the analytical workflow, particularly to be able to support that end user who just wants to be able to ask a question and get an answer without having to figure out what are all the tables I have to join.
[00:40:22] Unknown:
Or not even ask the question. Just get the answer. Why ask questions? I've seen AI machine learning evolve over the last 20 plus years, and I divide it into 3 buckets. There is a lot of AI machine learning designed to do the data transformation, which is not part of the BI tool, although we all have some basic functionality around it. And there's dedicated tools if you need to from turning unstructured data sentiment analysis all the way to dealing with very messy data and making it organized. Let's put that aside. 2nd is real time decision making transactional tools that I used to use in my cybersecurity days when in real time at a nanosecond speed, you have to decide what to do. Put that aside as well. That's a very different set of technologies.
I used to build some. I know how we look. It's a different discipline. And 3rd, there's machine learning and AI designed to help someone make decisions better, have better answers, sometimes without asking the questions, get deeper insights sometimes without realizing we need to. I believe that is analytics and that is part of BI, part of what we do every day. And I will divide that into 2 buckets. Those that come out of the box and we have insights since from predictive power to explanation, to cause analysis, to linear regression, to focusing, etcetera.
Natural language, AI and LG and LP and LQ, lots of fun stuff. And those that people have experts, data scientists or experts experts in their domain. For example, in the medical space to look at an image and say, cancer or not cancer. Or we work with with a lot of museums and cultural arts institutions. What is the optimal pricing you should ask for a ticket because it's actually a donation. You can ask for any amount what the what is the right amount to us. And here, data scientists should build the analytics on the side, but in mind you deploy it inside a tool like Sisense because there's no difference between a machine learning spitting out a recommended price to a forecast or a linear regression, they all sit on the same data, enjoy the same context layer, the same user context, the same visualization.
So I view that and the funnel type as things that are part of behind the analytics products just developed by the companies or by the data scientists in companies.
[00:42:56] Unknown:
As far as the kind of enablement of allowing your customers to be able to incorporate AI into that analytical workflow, what are some of the utilities that you provide either to make the generation of those models turnkey and easy to do where you say these are the data points I'm trying to optimize, feed that into my down stream AI use versus I have my own model that I want to develop and train but I want to run it in process with Sisense to make sure that it's able to work with those datasets that are embedded into this workflow? Great question. And you just answered it in the question already. We have 2 very different approaches, like like everything we do. For the very sophisticated
[00:43:35] Unknown:
developers, data scientists, data engineers, we support third party languages, Python, SQL. I don't believe in a proprietary language, so we don't have a mock up language like some vendors do and so on because it's an open ecosystem and I want you to use the tools you already know, just give it the language to run on Sisense. For the built in AI to make the human users that are not savvy, more capable, it's all transparent. It's like magic. The AI just makes a suggestion. You drag and drop a a chart and it shows your prediction without asking it to show you. You type in English, you get an answer in English. So it's really the same 2 extremes.
Very savvy people speaking the language they know, which is in this case, R, Python, SQL. And the end users, it's magic. They don't need to understand it. We just get answers.
[00:44:29] Unknown:
And that's the way it should be. I think the middle is not here, not there. In your work of helping to shepherd the Sisense product and working with your customers and end users, what are some of the most interesting or innovative or unexpected ways you've seen the Sisense platform used?
[00:44:45] Unknown:
I'm amazed every time at unexpected ways to use it. I have seen during COVID, a certain government use our technology to decide which ventilator to send to which ZIP code because we didn't have enough equipment in the country and they had to optimize for endless parameters. I have seen the use case in health where it saves lives again and again. We have a state of Indiana, I believe it was, used Sisense to build an application for donations. It increased by 300% the amount of donations people do, not for money, for body parts after a loved 1 dies and they give away their, you know, body parts to save other lives.
300% increase in donations of human body parts to save lives, purely thanks to analytics. I have seen Philips using Sisense to save unneeded anesthesia, when a child goes through a CT or an MRI scan. Apparently, they put an STIGI or 2 of them so we don't move around because it breaks the scan in this big machine. And they use analytics to find which kids need it, which kids will behave, and that will save unnecessary anesthesia. The United Nation is using Sisense to help fight infectious diseases in Africa. I've seen so many use cases save lives and help humanity that I'm actually, you know, I'm proud to do good in the world and to help people. And, yes, we have the usual sales marketing, revenue generation, inventory management use cases, But it's the others, helping the teachers and the doctors and, you know, the world environment and fighting COVID and so on that excites me and makes me sleep well at night and proud of what we do.
[00:46:32] Unknown:
And in your experience of leading this company and working with your customers, what are some of the most interesting or unexpected or challenging lessons that you've learned in the process?
[00:46:41] Unknown:
We are getting to a point in technology, which is quite scary that technology is not about the neck. It's imagination and culture. That was not the case up until a few years ago. I believe art is about to be there. Soon, art will not be about the ability to draw, but the ability to imagine what you want to draw, then the AI will draw it for you. So that's a fundamental shift that is happening in front of us, and it's not obvious. So that's 1. Number 2, I find that organizations are sitting on a gold mine of data that they can monetize and turn into value if only they wanted to.
People don't realize they sit on a gold mine. Imagine me telling you right now that under your churn, there's a big packet of gold and you're ignoring it. That's how much data is out there waiting to be leveraged to do good to people, businesses, and customers, and employees, and health, and everything. That's kind of a unappreciated asset that is all around us. So that's the second 1. And the third 1 is maybe the cloud from being something everyone tell me I'm crazy to offer them to asking me, why don't we run it on your cloud? That's a watershed moment that happened a few years ago, and the industry at last is there.
That changes many, many things because you can do things faster and cheaper on the cloud. So those are some examples I've been surprised to see the change.
[00:48:12] Unknown:
You touched on this a little bit earlier, but what are some of the cases where Sisense is the wrong choice?
[00:48:18] Unknown:
So the 2 areas I would not use Sisense. 1 is if you just want to build data pipelines. I believe every analyst company claiming to be a data pipeline company is is already a problem to begin with. Use dedicated tools to build the pipelines you need and then put the analytics on top. I have the ability to do data prep, but if you need a dedicated product, go and get 1. Number 2 is generic internal dashboards that are a dime a dozen and everyone can do. There's many ways to do it. If you need to embed analytics in externally facing applications or internally facing applications to your employees in a large enterprise, what have you, or to embed analytics in productivity tools like Slack or PowerPoint. Again, what whatever it is, that's a significant place. We cannot value APIs, developers, people that want to leverage AI for mere mortals and human beings, not for BI experts.
That's where we add value. And that's where we add value these days.
[00:49:18] Unknown:
As you continue to build and grow the platform and company, what are some of the capabilities that you're looking toward for the near to medium term or any projects that you're excited to dig into?
[00:49:28] Unknown:
Something we just announced is the next generation multi tenant capabilities. What we found is that most companies allow you to control entities using the analytics. Maybe you can control permissions, maybe the color. We built the ability to granularly manage every feature, every function, every model, every permission, every look and feel by a tenant in your organization. Could be a department, an employee, or a customer. That was a really complex engineering project that is now out there and is already valuable to customers. 1 we are working on is the next generation of APIs that allow you to come as a developer and get value overnight without even spending a day to get the first value. We're very excited around that. And a lot of AI, both AI for Miyamoto's that don't know analytics and data and AI for the data experts who know a lot of analytics and data and can use those tools to be innovative.
So those are things that excite me.
[00:50:35] Unknown:
Are there any other aspects of the work you're doing at Sisense or the overall space of business intelligence and embedded and ubiquitous analytics that we didn't discuss yet that you'd like to cover before we close out the show?
[00:50:46] Unknown:
Maybe the 1 thing is that I see more and more organizations and children from this economy monetizing for data into revenue streams, which is quite interesting. So versus using data for to operate your business is using data to make your offering to your customers more rich and appealing. And then reducing data to open a new revenue stream by selling data products and data services. And in this economy, when people can find out they can provide added value and make money by leveraging the datasets they sit on, that's quite appealing to them. And everyone win. Their customers get more value, their company gets more value, and their data experts get to do become more important because data was always a cost center in organizations.
That's becoming a revenue center in some organizations, which is quite interesting.
[00:51:40] Unknown:
Well, for anybody who wants to get in touch with you and follow along with the work that you and your team are doing, I'll have you add your preferred contact information to the show notes. And as the final question, I'd like to get your perspective on what you see as being the biggest gap in the tooling or technology that's available for data management today.
[00:51:57] Unknown:
Perfect. So first, if anyone wants to reach out, go to sisense.com, s I s e n s e. Or if you want to reach out with me directly, LinkedIn, Amir, a m I r, last name, Ored, o r e d. I'm happy to take your note. And then in terms of the biggest gaps in the industry, I believe that still today, 80% of the humans are not enjoying the power of modern data and modern analytics and modern AI. That's a gap. That's a mistake that we should all think about kind of in our daily occupation. And second, I believe that in organizations, the power of AI machine learning is still not understood widely enough by the executives to be leveraged to make the impact it can make.
And that's, education, knowledge,
[00:52:49] Unknown:
DNA, culture, imagination gap more than technology gap. It is not a technology gap. Well, thank you very much for taking the time today to join me and share the work that you and your team are doing at Sisense and some of the ways that you're working toward making analytics more accessible and available and understandable for people both in and outside of organizations. And I hope you enjoy the rest of your day. Thanks for having me. I enjoyed it.
[00:53:19] Unknown:
Thank you for listening. Don't forget to check out our other shows, podcast.init, which covers the Python language, its community, and the innovative ways it is being used, and the Machine Learning podcast, which helps you go from idea to production with machine learning. Visit the site at dataengineeringpodcast.com. Subscribe to the show, sign up for the mailing list, and read the show notes. And if you've learned something or tried out a product from the show, then tell us about it. Email hosts at data engineering podcast.com with your story. And to help other people find the show, please leave a review on Apple Podcasts and tell your friends and coworkers.
Introduction to Amir Orad and Sisense
Amir's Journey in Data and Cybersecurity
Overview of Sisense Platform
Use Cases and Audience for Sisense
Evolution of Business Intelligence
Self-Service Data Democratization
Role of Low Code and No Code Tools
Unique Capabilities of Sisense
Design and Architecture of Sisense
Integration and API-First Approach
Onboarding and User Workflow
Machine Learning in Analytical Workflow
Enabling AI in Sisense
Innovative Uses of Sisense
Lessons Learned and Challenges
When Sisense is the Wrong Choice
Future Capabilities and Projects
Monetizing Data and Closing Thoughts